Medical AI: The Challenges of Assessing Unseen Patients: Exploring the Potential and Limitations of Artificial Intelligence in Remote Patient Diagnosis
In the field of healthcare, the use of artificial intelligence (AI) has gained significant attention in recent years. AI algorithms have been developed to assist physicians in making personalized treatment decisions, especially in complex conditions such as schizophrenia. However, a recent study has shed light on a critical challenge that these algorithms face when presented with new, unseen data sets. Despite their initial success in predicting treatment outcomes, AI models often fail to adapt and perform accurately in diverse scenarios.
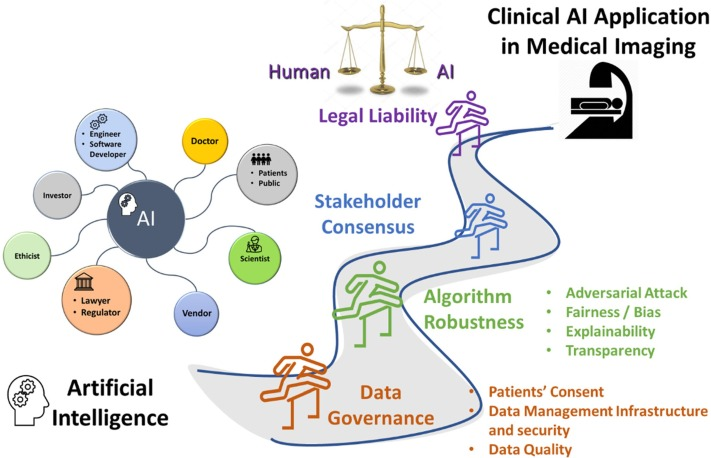
The Role of AI in Precision Medicine
Precision medicine is a rapidly evolving approach in healthcare, aiming to tailor treatment plans according to individual patients’ needs. It relies on the analysis of large data sets and the use of AI algorithms to detect patterns and predict treatment responses. Such algorithms have become central to precision medicine, offering the potential to optimize patient care and improve outcomes.
The Limitations of AI Models
A study published in Science has revealed that AI models used in psychiatric-prediction fail to adapt well to new data sets. The research team, led by psychiatrist Adam Chekroud from Yale University, conducted an analysis of five clinical trials involving participants diagnosed with schizophrenia. The algorithm used in the trials showed high accuracy in predicting treatment outcomes within the sample it was trained on. However, its performance drastically declined when applied to subsets of the initial sample or to different data sets altogether.
Testing Algorithm Accuracy
To assess the accuracy of the algorithm, the researchers trained it on data from the clinical trials and compared its predictions to the actual outcomes recorded in those trials. The results showed promising accuracy when the algorithm was tested within the trials it was developed on. However, when the algorithm was tested on new subsets of data or different trials, its predictions became inconsistent and resembled random outcomes.
Generalizing Algorithm Performance
The study’s authors emphasize the importance of rigorous testing to ensure the reliability of clinical prediction models. Only a small percentage of such models undergo validation on samples other than the ones they were developed on, according to a systematic review of psychiatric outcome prediction models. This lack of comprehensive testing poses a significant challenge in the field of medical AI.
Adam Chekroud compares the development and testing of AI algorithms to drug development. Just as drugs undergo rigorous testing at various stages, AI algorithms should be subjected to stringent validation to ensure their effectiveness. Building and testing algorithms should be an ongoing process, rather than a one-time endeavor.
Implications for Precision Medicine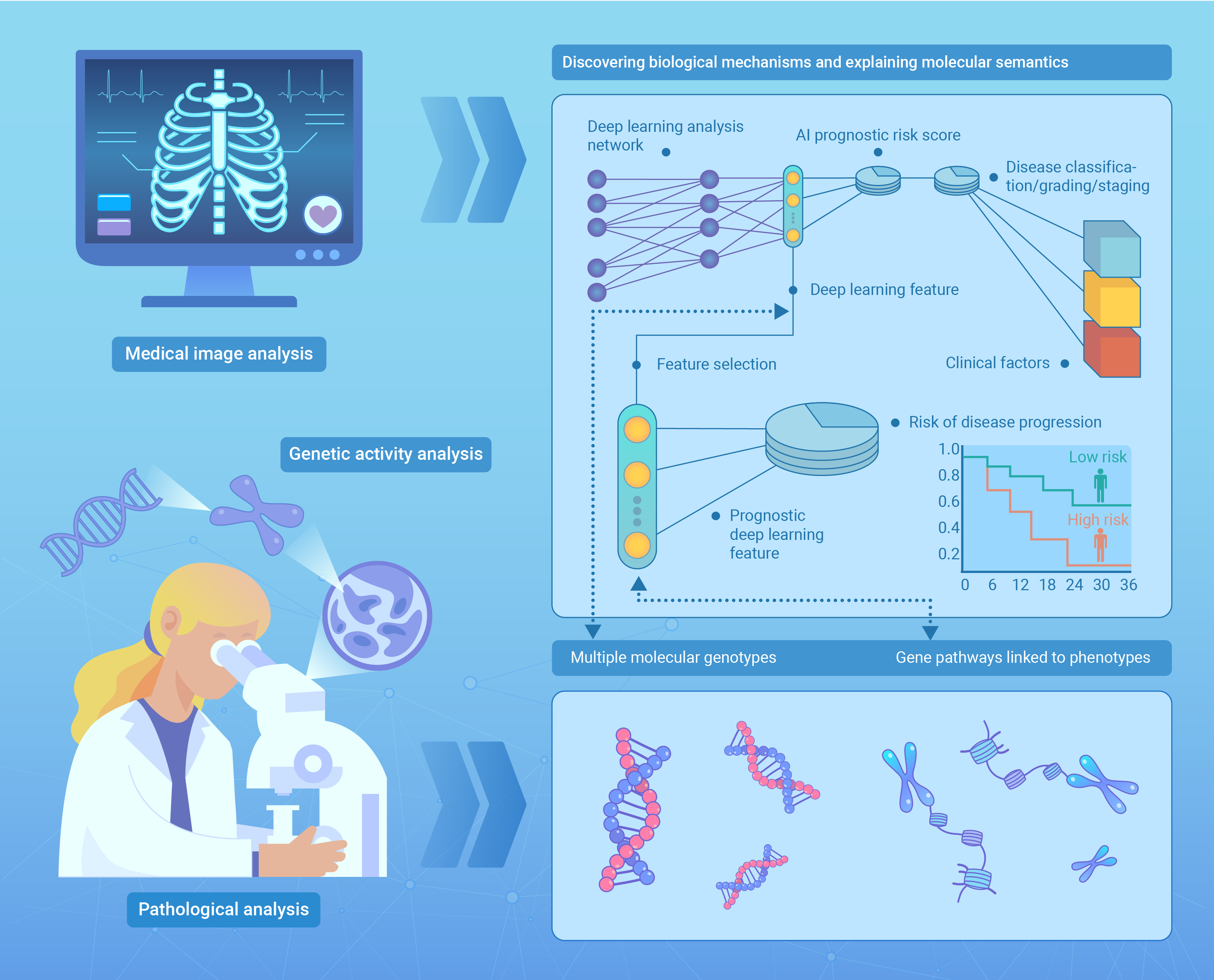
The findings of this study have significant implications for precision medicine. The inability of AI models to adapt to new data sets limits their applicability in real-world scenarios. Precision medicine aims to provide personalized treatment plans based on individual patient characteristics, but if the algorithms fail to perform consistently across diverse cases, the potential benefits of this approach may be compromised.
The Need for Further Research
While this study sheds light on the limitations of current AI models, it also highlights the need for further research in the field. Developing algorithms that can adapt and perform consistently across different data sets is crucial for the advancement of precision medicine. Researchers and developers must focus on improving the testing protocols and validation processes to ensure the reliability and accuracy of AI algorithms.
Collaborative Efforts in the Field
Addressing the challenges faced by medical AI requires collaborative efforts from researchers, healthcare professionals, and technology experts. By working together, they can develop more robust algorithms and establish standardized testing protocols. Open communication and data sharing between institutions and researchers can facilitate the creation of larger, diverse data sets that better represent the complexity of real-world scenarios.
Ethical Considerations
In addition to the technical challenges, ethical considerations must also be taken into account when deploying AI algorithms in healthcare. Patient privacy, informed consent, and the potential biases embedded in the algorithms are all important factors that need careful attention. Transparency and accountability in the development and implementation of AI models are crucial to ensure patient trust and the ethical use of these technologies.
The Future of Medical AI
Despite the current limitations, the potential of medical AI remains promising. As technology continues to advance, there is a significant opportunity to overcome the challenges and develop more accurate and adaptable algorithms. Continued research, collaboration, and the integration of AI into clinical practice can revolutionize healthcare, leading to improved patient outcomes and more personalized treatment approaches.
Conclusion
The study on medical AI’s limitations in assessing unseen patients highlights the need for robust testing and validation protocols. While the current algorithms may show promising accuracy within specific data sets, their performance drops significantly when faced with new scenarios. Collaborative efforts, ethical considerations, and ongoing research are essential to overcome these challenges and fully harness the potential of AI in precision medicine. As the field continues to evolve, the future of medical AI holds great promise in revolutionizing healthcare and improving patient care.